Joel Oskarsson
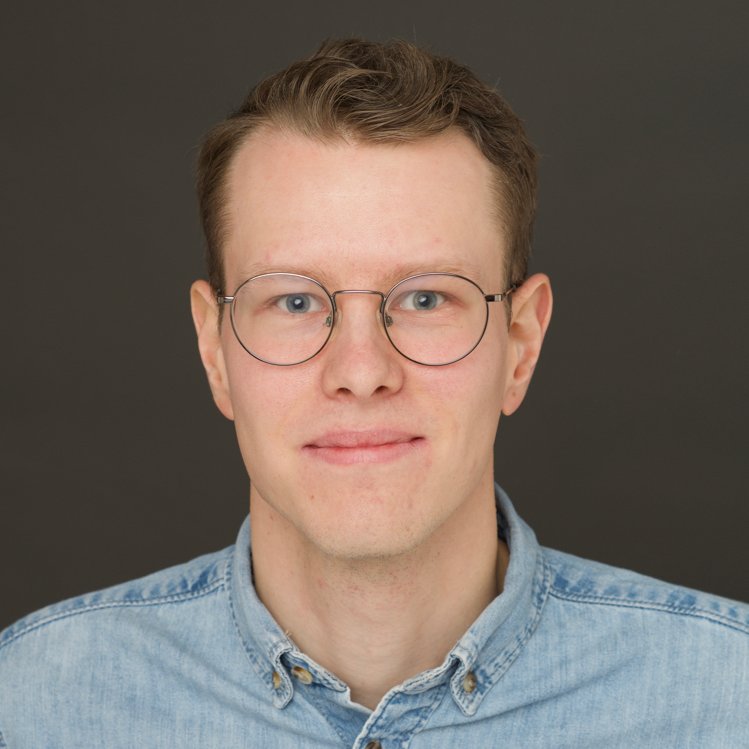
I am a PhD-student at the Division of Statistics and Machine Learning, Department of Computer and Information Science, Linköping University, Sweden. My main supervisor is Fredrik Lindsten and co-supervisors Per Sidén, Tomas Landelius and Jose M. Peña. I am an affiliated PhD-student within the WASP program.
In my research I work with probabilistic machine learning methods for modeling data with spatial and temporal dependencies. I am motivated by applications of machine learning to earth system modeling, such as weather forecasting and climate modeling. Part of my research is in core machine learning method development, where unique challenges from earth science act as an underlying motivation. These challenges include irregular observations, high-dimensional data, complex temporal dynamics and the need for accurate uncertainty estimates. I also enjoy developing methods specifically for earth science applications, tailoring the machine learning methods using domain knowledge to solve relevant problems in new ways.
I got my masters’s degree in computer science and engineering from Linköping University in 2020. In 2018-2019 I spent a year as an exchange student at ETH Zurich, Switzerland.
My CV is available here.
Current interests
These are some things that I am interested in and/or work on at the moment. I try to keep this somewhat up to date.
- Spatio-temporal data analysis
- Machine learning for modeling weather and climate
- Modeling continuous time signals using deep learning, Neural ODEs
- Machine learning on graphs
- Bayesian modeling on graphs, Graph GPs, GMRFs
- (Spatio-) Temporal graph neural networks
News
Dec 10, 2024 | I attended NeurIPS 2024 in Vancouver, Canada. Had a blast presenting our paper on probabilistic weather forecasting and meeting lots of amazing people! |
---|---|
Nov 7, 2024 | I gave a talk in the RISE Learning Machines seminar series, titled “Frontiers in machine learning for weather forecasting”. A recording is available on youtube! |
Oct 7, 2024 | New preprint: “Continuous Ensemble Weather Forecasting with Diffusion models” |
Oct 3, 2024 | Our paper “Probabilistic Weather Forecasting with Hierarchical Graph Neural Networks” has been accepted to NeurIPS 2024 as a spotlight! |
Aug 29, 2024 | I presented our work on probabilistic weather forecasting at the “Large-Scale Deep Learning for the Earth System” workshop in Bonn. Slides are available here. |
Aug 22, 2024 | I had the great pleasure to spend a week at ECMWF in Bonn as a visiting researcher. |
Aug 19, 2024 | New preprint together with collaborators from UCL and UKAEA: “Uncertainty Quantification of Pre-Trained and Fine-Tuned Surrogate Models using Conformal Prediction”. |
Jul 4, 2024 | We were interviewed by SVT (Swedish public service television) about AI weather prediction, ensemble forecasts and our research. Link to article + video (in Swedish). |
Jun 17, 2024 | Workshop paper “Valid Error Bars for Neural Weather Models using Conformal Prediction” accepted to the Machine Learning for Earth System Modeling workshop at ICML 2024. |
Jun 10, 2024 | New preprint on “Probabilistic Weather Forecasting with Hierarchical Graph Neural Networks”. Code is available on github, for global forecasting and limited area modeling. |
May 7, 2024 | I attended the ESA-ECMWF workshop “Machine Learning for Earth System Observation and Prediction” in Frascati, Italy. Had the pleasure to present our work on probabilistic weather forecasting (slides) and a community poster about Neural-LAM. |
Apr 28, 2024 | I am visiting Zürich for a week for research collaboration. I will also give two talks related to our work on neural weather prediction:
|
Apr 17, 2024 | I gave a talk for the CRUISE group at UNSW titled “Graph-based Machine Learning for Spatio-Temporal Data, with Application to Traffic and Weather Forecasting”. |
Mar 5, 2024 | I had the pleasure to visit the Department of Earth Sciences at Uppsala University and give a talk about our work on neural weather prediction. |
Jan 11, 2024 | I gave a talk about Neural LAM weather models at the webinar “Deep Learning for Weather-Based Power Prediction”, organized by IEA Wind Task 51. A recording is available on youtube and my slides can be found here. |
Oct 30, 2023 | I am visiting the Sustainability and Machine Learning Group at University College London throughout November (until 2/12). |
Oct 10, 2023 | I had the pleasure to give a talk at the Danish Meteorological Institute about our work on Neural weather prediction for limited area modeling. Slides are available here. |
Oct 2, 2023 | New preprint on “Graph-based Neural Weather Prediction for Limited Area Modeling”. Now also accepted to the Tackling Climate Change with Machine Learning workshop @ NeurIPS 2023! Code is available on github. |
Sep 4, 2023 | I attended the very exciting workshop Large-scale deep learning for the Earth system (webpage) and presented some ongoing work in collaboration with the Swedish meteorological and hydrological institute. Slides are available here. |
Jun 22, 2023 | I presented our work on Bayesian Learning on Graphs using Deep Gaussian Markov Random Fields at the NORDSTAT conference. Slides are available here. |
Jun 2, 2023 | Together with great collaborators at the Division of Vehicular Systems I have two papers on trajectory prediction accepted for publication:
|
May 10, 2023 | I presented my half-time-PhD seminar on “Graph-Based Machine Learning for Spatio-Temporal Data”. My slides are shared here. |
Feb 10, 2023 | New preprint: “MTP-GO: Graph-Based Probabilistic Multi-Agent Trajectory Prediction with Neural ODEs” |
Jan 20, 2023 | Our paper “Temporal Graph Neural Networks for Irregular Data” has been accepted to AISTATS 2023! Code is available on GitHub. |
Nov 14, 2022 | Together with the WASP graduate school I spent a week in Helsinki, visiting Aalto University and the Finnish Center for Artifical Intelligence. |
Jul 17, 2022 | I attended ICML 2022 in Baltimore, US |
Jul 4, 2022 | Conference paper + workshop paper accepted to ICML 2022 (Read More) |
Jun 13, 2022 | I attended the Nordic Probabilistic AI Summer School in Helsinki, Finland |